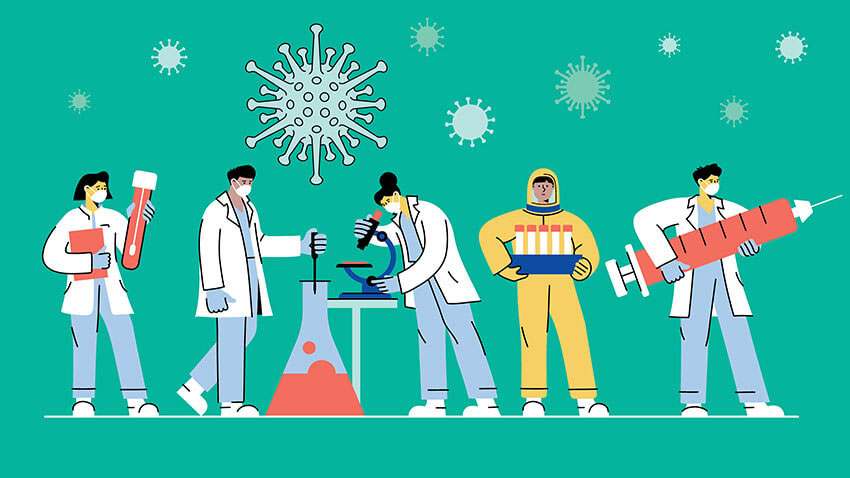
Analyzing the Covid-19 Vaccine Distribution Method and Experience
Analyzing the Covid-19 Vaccine Distribution Method and Experience
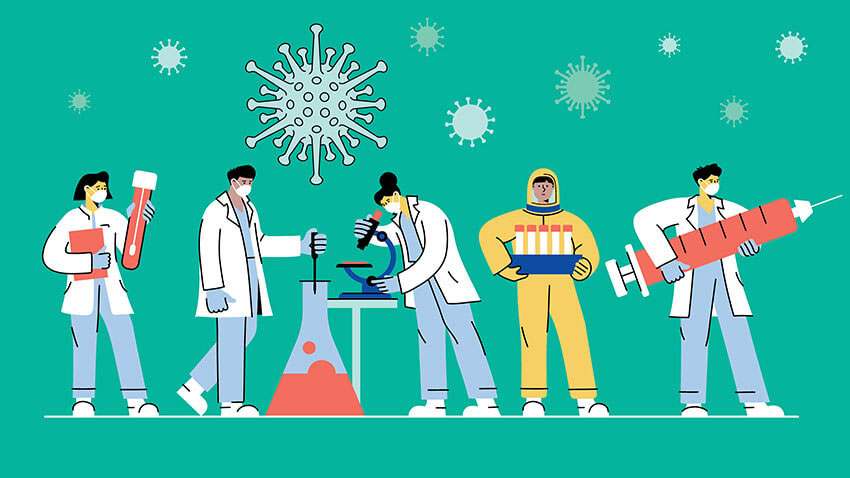
Introduction
As the Covid-19 vaccine begins to roll out for those who qualify for the initial distribution, the country as a whole is still seeing spikes and surges of cases, as well as high occupancy levels at hospitals and ICUs. The LA Times reported that “one hospital in southwest Los Angeles, Memorial Hospital of Gardena, is at 320% occupancy [and that] the 172-bed medical center has been in various levels of “internal disaster status” since March.” That said, as the vaccine becomes more available to the general public, it is important to understand what type of analytics can help us bring these shocking statistics down and how we can understand if distribution plans are effectively working.
Discussion
In evaluating the best way to roll out the vaccine, Accenture put together a list of relevant information to be collected for analysis. So what are the relevant data and statistics that go into a successful roll out? The first step in planning is forecasting and this is done by collecting data on a given population, this can be conducted at the county, city, state or regional level; relevant data in terms of the vaccine will include age and level of disease present in that population. From that information, distributors can decide where to locate dispensing sites and the supply necessary at each dispensing site. Once distribution starts, the next step will be adjustments which can be aided through artificial intelligence and machine learning. This would simply analyze the forecasting method used in the initial step and improve upon it so that new distribution centers are better prepared than the first ones. The final step is to evaluate the success of distribution which will vary based on the distribution leader, however, because the goal is to vaccinate as many people as possible and as quickly as possible, this will look at tiers that had the most waste in terms of vaccines and tiers that did not have enough vaccines to meet their demand.
One of the main concerns when it comes to vaccine distribution, on every level including the country level, is equality in distribution. An interesting point of data is that “as of 15 November 2020, several countries have made premarket purchase commitments totaling 7.48 billion doses, or 3.76 billion courses, of Covid-19 vaccines from 13 vaccine manufacturers. Just over half (51%) of these doses will go to high income countries, which represent 14% of the world’s population.” This brings up questions of income disparity as it relates to population health and is something that can also be tracked through analytics.
Conclusion
As accessibility to the Covid-19 vaccine increases, we highly encourage you to consider getting vaccinated. The policies and practices in place in this country make the vaccinations very safe, in fact,“the US has the best post-licensure surveillance system in the world making vaccines extremely safe and not getting vaccinated puts yourself and others at risk.” There is a lot of hesitation and concern when it comes to the vaccine which will make people less likely to get it on their own. To encourage you to get it as soon as it is made available to you, we are sharing the experience of Adiba Mobin, a UT Southwestern employee who has received both doses of the vaccine:
“I received the first dose of the Pfizer COVID-19 vaccine on December 23, 2020, just 9 days after the initial vaccine rollout in Texas. I did not take any medication (Ibuprofen, Acetaminophen, etc) before getting the vaccine. A few hours after receiving the first dose my arm became sore at the injection site and the soreness soon spread to my entire upper arm (shoulder to elbow) so I took some Tylenol for the pain which helped with the pain. Within the next 24 hours the soreness had vanished and I had absolutely no other side effects.
I received the 2nd dose on January 12, 2021, exactly 21 days later. This time I went prepared with a painkiller 30 minutes before getting vaccinated. The nurse administering my vaccine encouraged me to stay ahead of the possible side effects by taking Aleve or Tylenol specifically, as Ibuprofen might not be as effective. Once again, about 2-3 hours after receiving the second dose I had arm soreness at the site and at 5 hours post vaccination I could feel a headache coming on. At 8 hours post I took 1 extra strength Tylenol. The following day at 24 hours post-vaccination there was still arm soreness and a lingering headache but I did not take another Tylenol until 32 hours post vaccine, to help with some very minor muscle aches and arm soreness. All of my side effects were extremely manageable and nothing that took me away from my work for the day.”