Analyzing Alzheimer’s
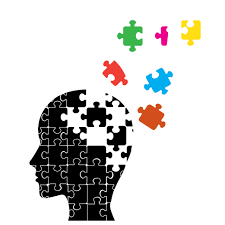
Analyzing Alzheimer’s Disease
Authored by Ayesha Rajan, Research Analyst at Altheia Predictive Health
Introduction
Alzheimer’s disease is a progressive disease that sees cells and cell connections die resulting in the loss of memories and mental function. It is a condition that affects 5 million Americans, with projections to affect 14 million people by 2050 and while there are medications to help treat symptoms, there is no cure. When trying to battle diseases without a cure, the best thing to do is to look at prevention and applying data science to that process can improve our understanding of how mitigating factors can benefit us individually. It can also help scientists create targeted gene therapies to help those affected by Alzheimer’s.
Discussion
Currently, a lot of the research being done in regards to Alzheimer’s disease revolves around genetics because of the genetic expressions and patterns that seem to be consistent in many of those that have the disease. To apply analytics to this knowledge, researchers at Icahn School of Medicine at Mount Sinai and Emory University formed a joint ventures with other research institutions to perform deep data analysis on mined DNA, RNA, protein, and clinical data. With this data they hoped to identify regulators and predictors of the disease in order to create targeted gene therapy treatments. What they found was that, though there are correlations between genetic expressions and Alzheimer’s, they were not strong enough to identify a singular cause. However, they did find that a protein called VGF plays an “plays an important role in protecting the brain against the onset and progression of Alzheimer’s disease.” Once they identified this connection, scientists could create a testing environment in which they “ramp[ed] up levels of the gene or protein in mice” and saw that those mice had a significantly lower risk of having Alzheimer’s or saw the progression of their disease slow down. Another gene therapy study out of Stanford University made a similar connection with the ApoE4 gene variant which is present in more than half of Alzheimer’s patients. Again, they did not find this to be a direct cause of the disease but prevalent enough that increased expressions of that gene increases the risk of Alzheimer’s. Studies like these are extremely important because understanding our genetic risk factors can help us understand how dedicated we should be to focusing on mitigating factors.
Conclusion
Alzheimer’s is a difficult disease to live with and, though genetic risk factors are unavoidable, living a healthy lifestyle can help prevent Alzheimer’s by lowering blood pressure and cholesterol, as well as lowering the risk of contracting diabetes, all of which have been connected to Alzheimer’s. Incorporating healthy foods and exercise into your lifestyle can help support these goals. Additionally, staying mentally active by continuing to learn and keeping up social connections has been shown to decrease the risk of Alzheimer’s as well. Finally, avoiding head trauma by taking precautions such as wearing a seatbelt, wearing a helmet and avoiding falls are all important steps you should take. Though we are discussing these prevention steps in terms of Alzheimer’s, they are all important steps to mitigating many other conditions.