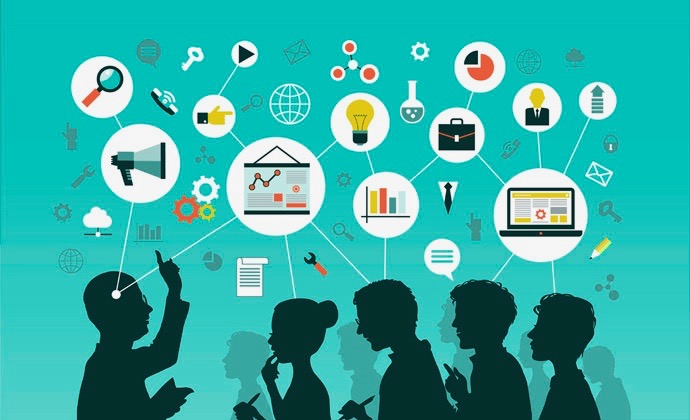
Small Communities Case Study
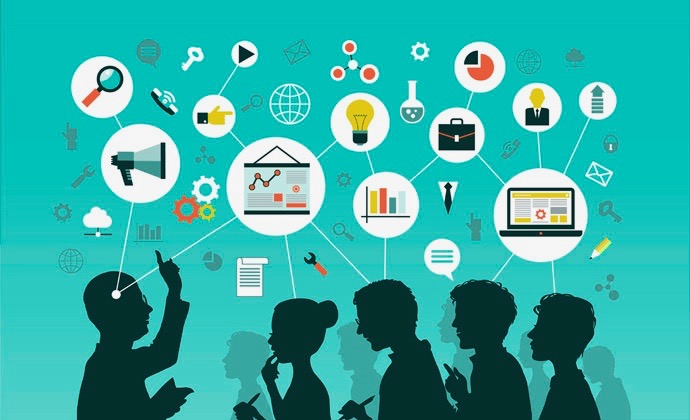
How Analytics Can Impact and Improve the Health of Small Communities (+ Case Study)
Authored by Ayesha Rajan, Research Analyst at Altheia Predictive Health
Introduction
Community health information can be very revealing; there is a lot to learn from the data of a specified community whether that specification be location, race, gender, occupation, age, or income bracket. However, these are relatively large communities and while that data is incredibly important, small community analytics can be even more targeted and actionable due to the ability to better communicate information to smaller groups.
Discussion
The biggest struggle in studying small communities is validity – many statisticians have argued that small community studies do not meet the benchmarks of a sample size to be referenced in other studies and this is a valid concern. However, if you don’t approach smaller studies as ones to be distributed, they can be especially impactful for their communities. Below, we’ve attached a case study from Cerner to demonstrate the importance of small community studies:
Cerner Case Study
Every year, approximately 735,000 Americans have a heart attack. There’s great interest in improving this number — and one of the ways we can contribute to that goal is by quickly identifying symptoms of and treating heart attacks. Troponin tests are commonly used in the emergency department (ED) to identify if a patient is experiencing a heart attack. In an ideal setting, the turnaround for a troponin test is about 35 minutes; most hospitals have a protocol setting of 60 minutes or less.
We recognized an opportunity for improvement with some of our clients around their troponin test rates. We pulled data on individual clients and compared it to industry wide data, and found that while some of our clients had fantastic numbers, others hadn’t had a focus group around this topic and there was room for improvement. If a hospital’s median turnaround time for a troponin test is 45 minutes, for example, that still means that approximately half their tests are taking longer than that.
Though there is currently no troponin test standard mandated by the Centers for Medicaid and Medicare Services (CMS), the turnaround time clearly impacts patient care. Think of it this way: The 25-minute difference in test results is akin to an ambulance arriving to pick up an individual with heart attack symptoms and then simply waiting in the driveway for over half an hour.
Conclusion
The study of the health of small communities can seem a little slow but actually has the potential to be extremely interesting! Small community studies can identify several localized issues ranging from issues in infrastructure to specified malnutrition to minor disease networks. Here at Altheia Predictive Health, we are specifically trying to make the research and benefits of big data accessible to small communities through our app. If you know a small company or organization that could benefit from our research, please send them our way!