Use of Analysis in Prediction and Prevention of Coronary Artery Disease
Ayesha Rajan, Research Analyst at Altheia Predictive Health
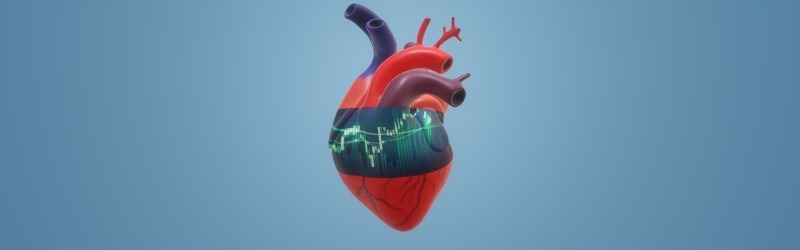
Coronary artery disease (CAD) is a chronic, comorbid condition that is usually the result of plaque buildup leading to limited blood flow. CAD is the leading cause of death and loss of productivity in the United States due to an aging population and globalization/ urbanization. It is expected to be responsible for over 20 million global deaths by 2030 (World Health Organization). As a result, CAD is clearly an issue that needs attention in terms of preventative care. While there currently exist many different prediction tools, most of them are severely lacking in the use of data points that are correlated with the presence of CAD.
The table in Figure 1 shows normal, at risk, high risk and highest risk (when possible) ranges for key points in a typical metabolic panel. If you could only look at a limited number of factors to predict and prevent CAD, these would be among the strongest points and are the basic data points included in most current predictive models. However, looking specifically at LDL cholesterol, as well as presence of diabetes or chest pain, cigarette-use, sex, race, and age can provide even more context and accuracy. One could cast an even wider net for more data points and include the results of a resting electrocardiograph, existing exercise induced angina or hyperlipidemia maximum heart rate, ST depression, slope of peak ST segment, and the total number of major vessels colored in fluoroscopy (Saxena).
Diastolic Blood Pressure | Systolic Blood Pressure | Total Cholesterol | Fasting Blood Sugar | BMI | |
Normal | <80 | <120 | <200 | <100 | 12-24.9 |
At Risk | 80-89 | 120-129 | 200-239 | 100-125 | 25-29.9 |
High Risk | 90+ | 130-179 | 240+ | >125 | <30 |
Highest Risk | 120+ | 180+ |
Figure 1: Key CAD Data Points in Metabolic Panel
Existing Analysis
The most prominent example of analytics in relation to CAD is the Framingham Risk Score which is a result of the Framingham Heart Study. The score is an algorithm that estimates a person’s 10-year risk of developing CAD in terms of low, intermediate, and high risk. It takes into account age, sex, presence of diabetes, smoking habits, systolic blood pressure, total cholesterol, HDL cholesterol and BMI or lipids. While the Framingham Equation is intensive and detailed, there are still a great number of factors that could improve its accuracy. For example, the prevalence of CAD varies in race populations: the corresponding age-adjusted prevalence of heart disease among whites, blacks, Hispanics, and Asians was 11.0%, 9.7%, 7.4%, and 6.1%, respectively (Virani). This is a data point with significant variance and using it as a prediction tool could improve the accuracy of the Framingham Equation or any other formula. Adding in other risk factors could also mean that the Framingham risk score could expand to include those who fall outside of the targeted 30-79 year age range or could include patients with diabetes, two groups the current scoring algorithm leaves out. The complexity in analyzing the symptoms and comorbid conditions related to CAD means that one in five patients are victims of misdiagnosis, further confirming the necessity to improve the existing analytics tools (Foote).
Where Can Analytics Take Us Next?
A topic that many people have heard of but equally as many people have not fully grasped is artificial intelligence. Artificial intelligence is the use of predictive models to forecast future events; in terms of CAD, computer programs look at and analyze all the data points available and come up with algorithms that have the strongest correlation variable possible. Currently, a company called Ultromics houses the EchoGo Core system which is an artificial intelligence technology that utilizes ultrasound images to identify disease. In its trials, its diagnostic performance yielded over 90% accuracy and halved the number of misdiagnoses compared to traditional clinical analysis (Foote).
Another direction we may see CAD prediction go into is genomics. It may not be a surprise to many people that CAD often runs in families; this fact indicates there may be data points in genomic profiles that can indicate the risk of having the disease. A study published by the Journal of the American Heart Association investigated the possibility that DNA could hold answers to predicting heart disease and concluded DNA methylation data could, in fact, aid in discovering high-risk individuals who were not classified as “at risk” by other studies, such as those with lower Framingham Risk Scores, which used metabolic panel data (Westerman).
Based on the preceding analysis, it appears the increased use of AI combined with access to a very broad data set is our best path to creating much more robust and accurate predictive models which can lead to earlier and more targeted interventions and better outcomes.
In a study published on NCBI, individuals who changed the following things about their lifestyle and diet also showed a decreased risk of contracting CAD: avoid smoking, increase physical activity, avoid being overweight, using healthy fats, eating fruits and vegetables, using whole grains, reducing, sugar and reducing sodium (Razzak). All of these things are also well-known components of a generally healthy lifestyle and mitigating factors for many other chronic conditions and disease other than CAD.
“About Cardiovascular Diseases.” World Health Organization, World Health Organization, 29 Sept. 2011, www.who.int/cardiovascular_diseases/about_cvd/en/.
Foote, Natasha. “Artificial Intelligence Technology Developed to Predict Heart
Disease.” Www.euractiv.com, EURACTIV.com, 30 Apr. 2020, www.euractiv.com/section/health-consumers/news/artificial-intelligence-technology-developed-to-predict-heart-disease/.
Razzak, Muhammad Imran, et al. “Big Data Analytics for Preventive Medicine.” Neural Computing & Applications, NCBI, 16 Mar. 2019, www.ncbi.nlm.nih.gov/pmc/articles/PMC7088441/.
Saxena, Kanak. “Efficient Heart Disease Prediction System.” ScienceDirect, 2016, www.sciencedirect.com.
Virani, Salim. “Heart Disease and Stroke Statistics—2020 Update: A Report From the American Heart Association.” AHA Journals, 2020, www.ahajournals.org/doi/10.1161/CIR.0000000000000757.
Westerman, Kenneth. “Epigenomic Assessment of Cardiovascular Disease Risk and Interactions With Traditional Risk Metrics.” Journal of the American Heart Association, 2020.