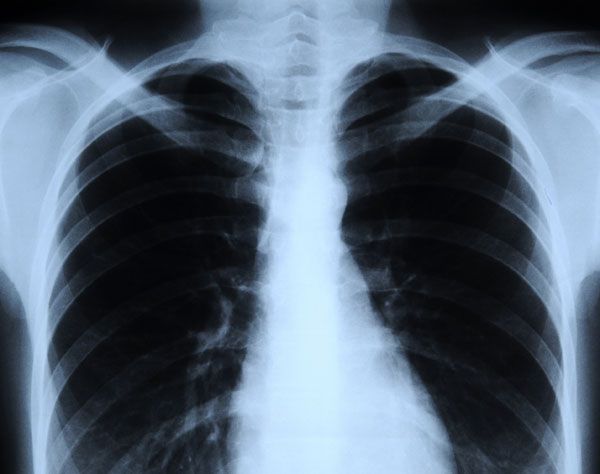
What Role Can Analytics Play in Imaging?
Authored by Ayesha Rajan, Research Analyst at Altheia Predictive Health
Introduction
X-rays are a key part of many treatment plans because of the valuable information they provide. However, they do come with a small increased risk of certain cancers. While this is not a worrisome point for most people who are having diagnostic imaging performed, it can be of concern for those patients that are monitoring symptoms and need more frequent imaging performed. This creates a demand for more efficient image reading techniques. Artificial intelligence methods have been very successful in image recognition and have become an important and useful tool in improving x-ray readings. Today we will look at the methodologies used in this process, as well as one of the companies leading the way in these endeavors.
Discussion
How: Applying AI and Machine Learning to imaging happens through intensive training of models. Engineers have to create incredibly specific parameters within their algorithms that tell models how to identify pixelated or 3-dimensional characteristics of abnormalities, such as tumors. Their algorithms are generally focused on finding flagged biomarkers and the methodology is generally supported by support vector machines and random forest. Learning architectures can also be supported by convolutional neural networks that map images and focus on the extraction of key figures/points. All of these methods increase the quality and sensitivity of image readings such that they are more accurate when being processed through an algorithm than they are when read by the human eye, i.e. a radiologist.
Who: CheXNeXt is an algorithm created by researchers out of Stanford University who sought to increase the accuracy of diagnoses of chest conditions by applying artificial intelligence and machine learning techniques to the imaging process. CheXNeXt works by training its dataset which consists of “112,120 frontal-view chest radiographs of 30,805 unique patients [and] using… automatic extraction method on radiology reports” before training its data. The training process “consists of 2 consecutive stages to account for the partially incorrect labels in the ChestX-ray14 dataset. First, an ensemble of networks is trained on the training set to predict the probability that each of the 14 pathologies is present in the image. The predictions of this ensemble are used to relabel the training and tuning sets. A new ensemble of networks are finally trained on this relabeled training set. Without any additional supervision, CheXNeXt produces heat maps that identify locations in the chest radiograph that contribute most to the network’s classification using class activation mappings (CAMs).” With these datasets CheXNeXt is able to accurately diagnose 14 chest-related diseases with more accuracy than a radiologist.
Conclusion
Artificial intelligence techniques have not yet made their way into the mainstream. However, initial research and testing suggests that the application of AI and machine learning can have an important impact on the diagnosis of many conditions picked up by x-rays. CheXNeXt is one of a few companies that is leading the way on this initiative and, hopefully, as time goes on we will see applications of this technology to x-rays in search of conditions such as bone cancer, digestive tract issues, osteoporosis and arthritis. Additionally, this is a hopeful step that researchers can reduce the need for repetitive x-rays by making diagnoses happen in a more efficient manner – one in which artificial intelligence supports a radiologist.