
Do Analytics Discriminate? Disparities in Algorithms Across Various Racial and Ethnic Groups
Authored by Ayesha Rajan, Research Analyst at Altheia Predictive Health
Introduction
Patients who come in with concerns about kidney function are always tested for their glomerular filtration rate(GFR). It is interesting to note, the GFR is factored into the Kidney Failure Risk Equation and other algorithms differently for African Americans but remains standard across all other ethnic and racial minority groups. However, across several other predictive health algorithms and risk calculators, racial differences in the metabolic panel are typically not considered. Given that different racial and ethnic groups have different predispositions to and progressions of diseases, this is an area that needs improvement. As the field of medicine moves towards analytics to predict patient risk, medication needs and other factors, it is important to consider the benefits of increased accuracy by taking race into consideration when creating algorithms, as well as the implications of biased algorithms.
Current Problem
In 2019, NBC reported on an algorithm written by Optum that was heavily biased. The algorithm was used to rank patients that would most benefit from a care program that aimed to manage responsible use of medication and to keep patients out of the hospital. In a group of 6,000 black patients and 44,000 white patients, only 17.5% of people recommended for the program were black despite the fact that the black patients had 26.3% more chronic conditions. Developers had to ask themselves why this was the case and found that their cost-based algorithm left behind black patients because of monetary biases – black patients spend $1,800 less per year than white patients with the same conditions. This means that black patients with the same or worse chronic conditions than white patients were left behind because of their inability to pay. Once Optum balanced that aspect of the algorithm, they saw the number of black patients recommended for the program jump from 17.5% to 46.5% (Gawronski). This example shows disparities in algorithms that we can not always anticipate but that still negatively affect certain racial groups.
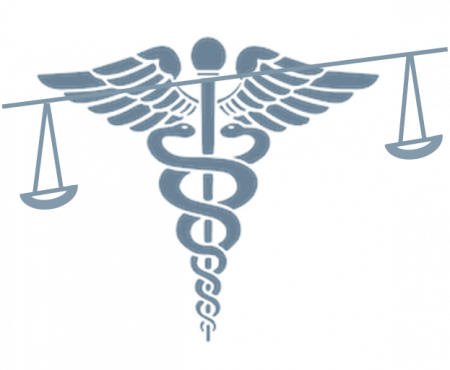
Analytics As A Solution
In October of 2019, George Washington University received a grant to study these types of disparities. The four-year study is still in its earliest stages, but it will help researchers better identify the relationships between race and disease prediction. Yan Ma, who is the Vice Chair of the Department of Bioinformatics at George Washington University, says that one of the biggest and easiest steps that can be taken to improve this situation is for large databases to include a patient’s ethnic group and/or race in their information. In fact, it’s surprising that many data bases do not already have this data. If they did, it would make machine learning and artificial intelligence even more powerful tools than they are currently (Kent).
The Centers for Medicare & Medicaid Services echo the same sentiment in their studies of using racial data to improve healthcare treatment for all patients, citing lack of clear and reliable data as the biggest roadblock to their research and the area most in need of improvement. Currently, Medicare uses (and has been using for quite some time) a method called geocoding to target at risk communities. Geocoding makes predictions about a person’s health based on the characteristics of the areas in which they live. It works in the way that many would like algorithms to account for racial differences; it takes into consideration the population health and trends of a community to make more targeted predictions about a person’s health. This method is limited because it is not exact and is highly dependent on areas that self-segregate. It does however lay out the groundwork for how a study could separate certain groups to identify their specific risk factors and general health.
Conclusion
While data science tools such as machine learning and artificial intelligence have significantly advanced the field of medicine, there are many people that are left behind because of biased equations. In order to best serve all communities it is important that healthcare providers and companies that are creating predictive algorithms take differences in racial predispositions into account and adjust metabolic and blood panels in the same way to provide the best care to each unique patient.
Works Cited
Gawronski, Quinn. “Racial Bias Found in Widely Used Health Care Algorithm.” NBCNews.com, NBCUniversal News Group, 7 Nov. 2019, www.nbcnews.com/news/nbcblk/racial-bias-found-widely-used-health-care-algorithm-n1076436.
Kent, Jessica. “Machine Learning to Uncover Racial Disparities in Healthcare.” HealthITAnalytics, 31 Oct. 2019, healthitanalytics.com/news/machine-learning-to-uncover-racial-disparities-in-healthcare.
Llanos, Karen. “Using Data on Race and Ethnicity to Improve Health Care Quality for Medicaid Beneficiaries.” CHCS, 2006, www.chcs.org/media/Using_Date_to_Reduce_Health_Disparities.pdf.